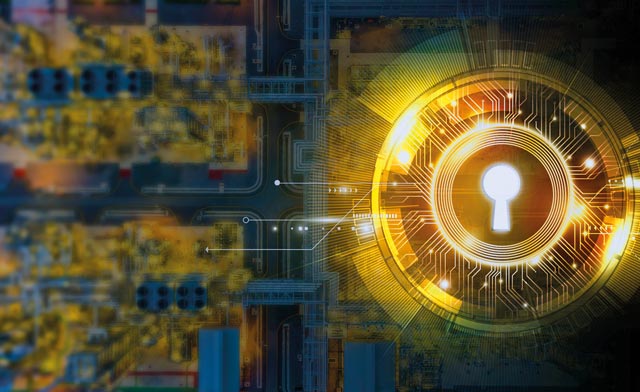
Predictive maintenance isn’t all predictive analytics, it’s about directing human attention to where it is needed, says Senseye chief technology officer Robert Russell
Long before the first predictive maintenance technology was developed, most engineers developed a special sense while working with and looking after “their” machines. This could have been called the original predictive maintenance system, as these experts used their knowledge and experience to pick up on and evaluate every change in the noises the machine made, every rattle and vibration. Very often, they just knew when something was about to go wrong and were able to intervene before any major damage was done.
The advent of more advanced technology does not mean that this expertise isn’t needed anymore. Our experience shows that a predictive maintenance system is much more accurate and efficient if it can tap into this pool of knowledge. It can learn to work with the engineers and provide the best possible support in the decision-making process.
Although zero downtime would be the ideal, it is an unrealistic expectation. All machines need to be maintained and repaired at some stage. It just becomes much more economical and efficient if this downtime can be planned and carefully managed.
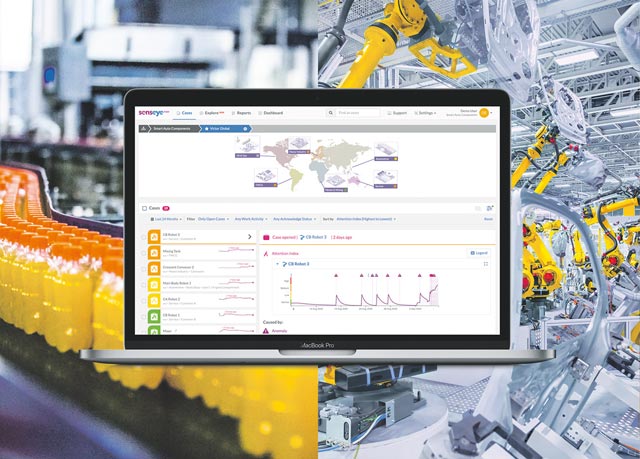
Experience supported by data
Industry 4.0 and smart factories create massive amounts of data: data that needs interpretation to be useful in any way.
In most industries, it is impractical to build a detailed and robust mathematical model specific to each individual machine, so most predictive maintenance systems work instead with limited data inputs from the factory floor evaluated against simple alarm levels. They might alert users when something is heating up or vibrating more, but they are unlikely to provide enough information to make a detailed diagnosis. That’s where the experienced engineer has to make the decision when to stop a machine.
Entry level predictive maintenance systems are designed to support the knowledgeable user, though most take the approach of needing a “digital twin” (specific model) of each asset. They accept a range of inputs and look for patterns or characteristic signals that indicate that there might be a problem. More advanced systems can forecast failures before they cause an unplanned shutdown. In many cases, and with sufficient data, they can even calculate the remaining useful life while moving away from the necessity of manually developing specific asset models.
The Attention Index
For predictive maintenance to succeed, Senseye believes the goal should be to minimise human involvement in filtering items of interest, to direct human expertise where it can be most powerful.
Moving away from manual model creation and maintenance, instead Senseye developed the Attention Engine to process the massive amount of data from the plant in order to predict how interesting emerging trends, anomalies, patterns and prognostic matches were likely to be to the operator. It thus created the Attention Index to rank where the maintainer should be focusing.
We have added a feature that allows the operator to evaluate every case and, with a touch of a button, indicate if it was useful or not. Over time, this teaches the system to notify the operator of the most pressing maintenance priorities, and not waste their time with low level information. The system is learning all the time without the need for extensive operator training or data scientist coding. If the Attention Index reaches the level of a warning, it also makes it clear why. The user can see the underlying problem before having to look at the detail, and can make decisions quickly.
Looking for patterns
Data analytics is about searching for patterns in the mass of incoming plant data. The patterns behind the Attention Index can be anomalies, trends or violations. Anomalies are periods of unstable data; trends are represented by gradual shifts in the baseline. Rules or thresholds, when broken, result in a violation, and can be specified by the user.
Although all these methods of analysis are fully automated, they are completely transparent to the user and can be tailored accordingly. Adding a predictive maintenance system should enhance existing monitoring systems and support their users, not replace them.
www.senseye.io | +44 (0) 845 838 8615