The impact on productivity of predictive maintenance based on sensors and advanced data analysis will become even more significant as computer programs that can learn for themselves continue to evolve, says SKF’s head of service, Ian Peverill.
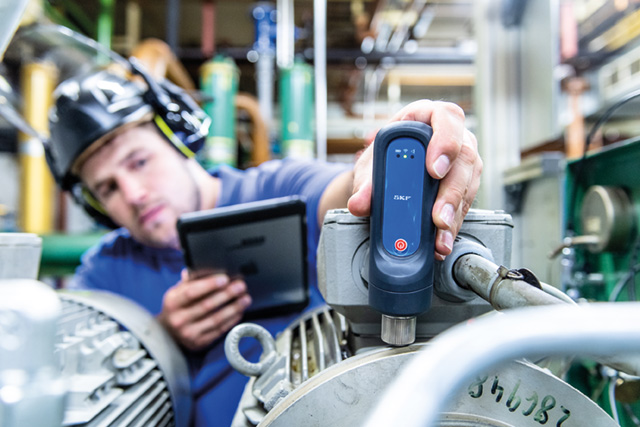
The ability to predict the exact remaining useful life (RUL) of any given machine or component could revolutionise the industrial world. Unscheduled downtime could be eliminated entirely, enabling organisations to be more productive, more efficient and more agile than ever before.
Despite the increasing sophistication of technology for monitoring the health of machinery, however, such predictions cannot yet be made reliably and accurately. Detecting a problem with a subsystem such as a bearing is one thing, but being able to determine how long it will last with pinpoint precision is quite another.
Data and how it is handled will be key factors in the development of systems for the prediction of RUL. A wide variety of wireless electronic devices such as sensors that can gather data on the health of machines or subsystems, and transfer it rapidly to the internet for analysis, already exist. The sheer volume of information collected by such sensor systems, however, can be overwhelming and its interpretation presents significant challenges.
Take the vibration data gathered from a bearing as just one example: how can that be linked with all the other process data – on temperatures, pressures, flows, running rates and so on – that a machine or plant might generate?
Machine-learning algorithms that enable the classification and interrogation of truly massive volumes of information will be of vital importance if the potential of condition monitoring is to be exploited fully. While conventional computer programs follow a set of hard-coded rules which specify exactly the steps they need to take in order to solve a problem, machine-learning algorithms can identify patterns in massive amounts of data. They can then apply what they have learnt to new situations.
The quality of insight a machine-learning algorithm can provide is proportional to the quality of the data it is fed. SKF has collected data from many millions of bearings, but this has to be prepared before it can be interrogated accurately and reproduced using such algorithms. Although only a small proportion of the data collected will be required for the machine-learning process to be effective, it can be difficult to identify the right information for the right application.
Moreover, data is often held in silos and sometimes in different formats, making it difficult to interrogate as a whole. Because of this, ways in which data can be labelled, stored, combined and classified must be developed.
At SKF, this work is being carried out in parallel with the development of effective methods for the interpretation and analysis of data in machine learning systems. Through the approach being refined, data is first used to establish that a problem exists with a component or machine. The analysis of this data then enables the exact nature of the problem to be defined, before appropriate remedial action, and the right time for that action to be taken, are determined.
Through machine learning, detection, diagnostics and prognostics will be carried out automatically. The rationale driving these decisions, together with the ways in which the machine is run in order to prolong its operating life, will improve continuously as increasing volumes of data are fed to the machine-learning algorithm.
Significant progress is being made and the rapid evolution of machine-learning technologies is, in turn, driving the development of new bearing and sensor technologies. While we may not yet be able to predict RUL precisely, we are getting tantalisingly close.
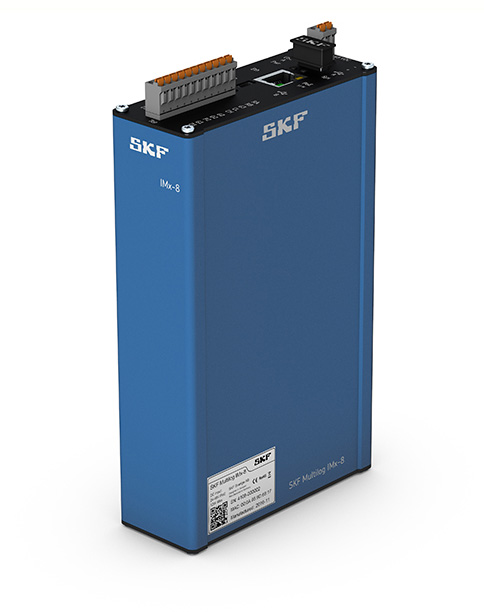
Further information:
Sian How, PR and brand awareness manager EMEA, SKF (UK)
T: 01582 496433 M: +44 (0) 7545903710
sian.how@skf.com